It won’t be entirely wrong to say that humanity runs on trust. Trust in people. Trust in the car that you drive, for it to not break down. Or that train that you’ve been taking to work and back. In the philosophical sense, ephemeral trust is not what strong institutions are built on. A trustworthy teacher is probably someone who knows what they are teaching. A trustworthy car mechanic knows where the problem is, just by hearing the sound that the engine makes. These trustworthy people exude quality. And that’s the same with data. You can trust data to get you to the insights that you want, only if your data is of high quality – without any unwanted errors, structured in a manner that your machine learning models can process it, and in the form that you have intended the data to be.
How can organisations achieve high-quality data to make informed, empowered, and data-driven decisions?
Before we get into the process of reaching the stage of that clean data, it’s important to understand the kind of inconsistencies that can creep into the data that organisation gather from various sources.
- Duplicate data
The biggest culprit in the process of data gathering is duplicate data. You might have duplicate data that can show high sales of a particular SKU just because of duplicate data. A non-structured approach to cleaning data might result in sales data being reported at a much higher rate than the actual one.
- Incomplete data
What’s worse than duplicate data? Incomplete data.
Imagine that your machine learning solution requires 8 parameters to come up with a proper predictive model. But your data is missing 3 such parameters. And if the weightage of these missing parameters is higher, you will get heavily skewed results, even if the model were to assume the missing parameters as NULL.
- Incorrect data
When you get data for one product, that was meant to represent another product, you have a major problem at your hands. One way to fix such a data issue is to go through thousands of lines of data in a data-set manually and rectify it. The other is to do so in a jiffy through technology, through artificial intelligence and machine learning.
- Inconsistent Data
This is an interesting one and requires an interesting approach. Do you have the same data representing different data points in your database? Does the data from one source show up as a source for data where it should not? Get the drift? Inconsistency in data can arise from seemingly lesser known reasons – data integration issues, assumptions, non-structured approach to data processing.
- Data downtime
There are times when your data sources don’t perform in the manner that you want them to. They could send partial data, incorrect data or even no data. Your data models must cater to handling such situations through data reconciliation and other such methods.
As per 2021 Global Data Management Research:
33% of companies feel their data of customers and prospects is incorrect
55% leaders don’t trust their own data
95% companies feel that poor data quality is affecting their business
50 of respondents feel that their CRM and ERP data is correct
60% time of data scientists goes into cleaning and preparing data
76% of data scientists do not view data preparation as an enjoyable activity
$3.1 trillion is the estimated business loss to companies in the US, due to bad data
How does bad data impact real-world scenarios?
Some scenarios that might play out owing to bad data can have huge negative repercussions in real-world conditions. Some of them are listed below.
- Outdated customer information, duplicate entries for customers, missing or incorrect contact details etc. which can lead to missed opportunities and revenues
- Missing emergency contact number for patients can result in not being able to get consent from the next-of-kin during medical emergencies
- Missing users’ demographic information can limit the ability to upsell/recommend customized and user centric services/products for eCommerce platforms
Consequences of poor data
Poor data does not come alone, it brings many problems to the business. It’s almost like bringing the cavalry in, only to slow the organisation down in the process of decision-making and getting proper insights,
- Sub-optimal utilisation of time, money and human resources, and creation of cost overheads
- Lower credibility of analytics and the accuracy of decisions made
- Negative impact on customer experience and trust
- Missed opportunities and revenue losses
No business is immune to data quality issues. If data is collected in an unorganised manner, bad data is bound to sneak in in hordes and become unmanageable over time. There is no quick fix for data quality issues, and such challenges must be addressed at multiple levels through implementation of an organisation-wide strategy, and not just a localised data strategy for one business function.
Creating an organisation-wide data strategy
- Data governance frameworks should be in place – Setup process, tools & identified owners for better enterprise data management
- Stop bad data from entering systems – Data entry applications should have mandatory fields that don’t allow users to bypass these fields. A data review policy will help in ensuring data cleanliness in this process.
- Perform data quality checks at the entry level – Setup data quality check at the entry levels and stop bad data from getting into data pipelines
- Perform regular data quality audits – Regular data quality audits by 3rd party organisations will ensure high governance practices in the organization
- Deployment and integration of data quality management tools in data pipelines – In most organizations, data is growing at high volumes and at a high pace, which makes it impossible to perform data quality checks manually – it is labour intensive, prone to errors and a waste of time and money, and more importantly, of human intellect. Organisations must identify and deploy data quality management tools in their data pipelines to ensure that data quality is maintained.
Meet the Data Sentinel – your one-stop solution for maintaining data quality
At Prescience we use Data Sentinel a data quality management tool to check data quality issues for various data sources such as SQL DBs, CSV, Excel and flat files. It is designed for various user personas such as Data Steward, Data Scientist, Data Engineer, etc., each having their own data requirements. Our Data Sentinel has a library of 200+ predefined DQ rules and allows for creation of more custom DQ rules based on organisations’ needs. Application users can pick and choose these rules based on their need and create rules that are required for their data sets.
In fact, users of our Data Sentinel can offload time consuming processes to the platform. We have seen time optimisation of up to 30-40%, time that can be used to focus on addressing customers’ problems.
The Data Sentinel generates various metrics on data quality such as total vs passed rules, a DQ score – which quantifies the data quality, higher the DQ score better the data quality. All this is brought to you through the power of artificial intelligence, machine learning and an understanding of your business scenario.
Rule checks can also be integrated with existing ETL pipelines, and can be triggered at a scheduled time or through an application-driven event.
Some common data quality rules that can help your organisation
- Duplicate value check – Ex. Checking duplicate customer emails
- Unique value check – Ex. Ensure that employee IDs are unique
- Mandatory fields, Null or missing value check – Ex. Missing customer contact info such as phone no., email ID, etc.
- Format consistency – Ex. Consistency in date or currency formats
- Range of allowed values in a column – Ex. City names where an eCommerce company operates its business. A number, in this case, would be a red flag.
- Last data refresh time from data source – Ex. Identify how fresh or stale the data is, based on the last time it was fetched
The Data Sentinel tool also allows customers to configure DQ Score bucket as per their business requirements.
An example of the DQ score bucket configuration
When DQ score goes below the defined threshold limit, the tool notifies the data owner/steward to take corrective actions on the data and make sure that data quality is high for analysis.
To know more about Prescience Data Sentinel tool and data management practices we follow, write back to us sales@prescienceds.com.
If you are looking for a starting point for your business, take advantage of our personalized FREE consultation workshop Sign up here.
Subscribe for regular updates on AI and Data Innovations, case studies, and blogs. Join our mailing list.
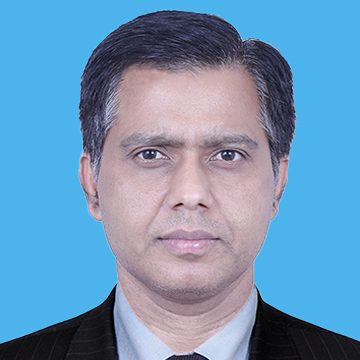
Umesh has more than 20 years of IT industry experience in designing and developing enterprise software products and SaaS applications. His areas of expertise are system and enterprise storage products and Ed-tech solutions. His favourite pastimes include playing tennis, reading technical articles and spending time with his kids… and coding.