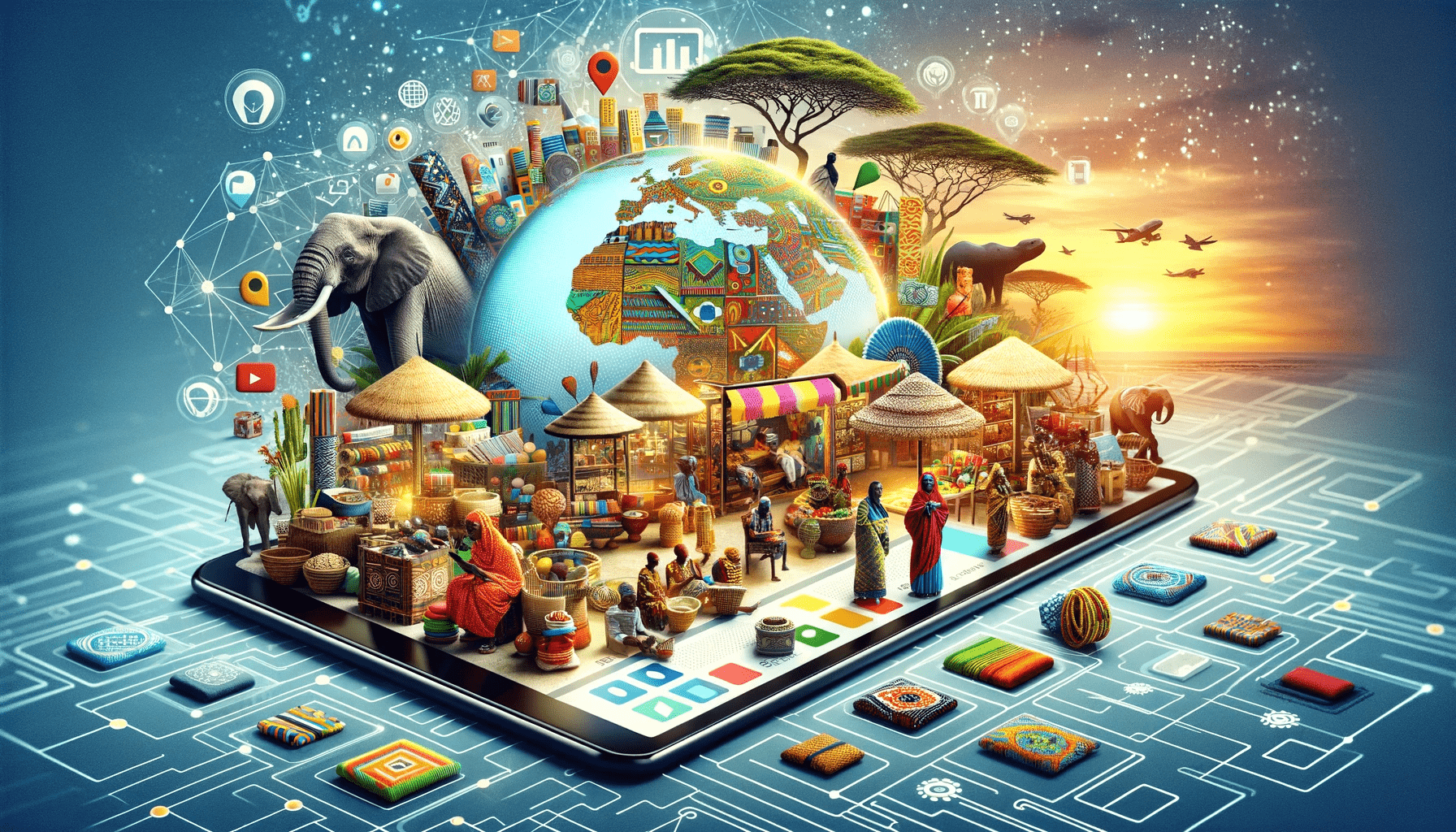
The company is the only B2C e-commerce platform built for the African mass market. They have 2 million customers who have placed over 13 million orders on their platform. Through a combination of digital channels and 50,000 agents across Kenya, the company serves over 800 million middle-to-low income consumers.
THE CHALLENGE
African consumers buy around 70% of their personal care products, food and beverages from over 2.5 million small retail outlets. In Kenya, around 77% of consumers purchase their goods from over 250,000 small retail stores.
Unlike these traditional retail outlets, the company offers its customers the ability to place orders through an agent’s catalogue or on the company’s website. This innovative model makes it easy for the under-served middle to low-income customers to conveniently receive high quality goods, that were previously unavailable to them unless they travelled to large stores in nearby towns and cities.
When a customer places an order, the company transports the required items from its major distribution hubs to its agents, who are typically local shopkeepers. Each agent covers 4 to 5 neighbouring villages. Customers pick up their orders from the agents. If there are items to be returned, then these agents collect the goods from the customers and send it back to the distribution hub for processing.
Considering their national reach, the hub and spoke business model and the growing scale of operations, the company incurred significant overheads due to process inefficiencies in their supply chain, manpower management and logistics. Their business users also wanted to eliminate the persistent problem of several products being frequently stocked out and the corresponding delays in re-ordering them. Another target for the business users was the reduction of the cost per drop metric for their end-to-end operations.
The company needed an IT partner to develop a comprehensive analytics-based demand forecasting system which would optimize their inventory, people and fleet management.
THE SOLUTION
The team of architects and data scientists from Prescience Decision Solutions analysed the preceding 3 years of the company’s historical data. This included various events such as the Covid pandemic, changes in the government etc. Third party market data was also incorporated into the study. The team also explored different economic scenarios, agent data, holiday data, consumer data, and marketing campaigns. The data preparation activities included data cleansing, missing data management, outlier handling etc. The different Stock Keeping Units (SKUs) were categorized into fast-moving, high-cost, novelty etc.
To accurately forecast demand at a product level, the Prescience team examined multiple variables including total sales, the average order value, number of customers, number of agents, average order per agent, promotions etc. Based on the available data and the existing business challenges, the team explored different models including the Linear Regression model, Random Forest model, XGBoost (Extreme Gradient Boosting) model, CatBoost model, ARIMAX (Autoregressive Integrated Moving Average with Explanatory Variable) model and SARIMAX (Seasonal Auto-Regressive Integrated Moving Average with eXogenous factors) model. A combination of two of these models accurately predicted the orders at an SKU level, and cumulative level. For selected SKUs, the Vector Autoregression (VAR) model was used and for other SKUs, the Moving Average model was implemented.
The Prescience team was also able to predict the SKU level demand and automate alerts for re-ordering products when the inventory levels crossed the minimum threshold values. With this new system in place, the previous lead time was eliminated as the company no longer needed to reach out to their suppliers when products were stocked out
The different technologies used for this engagement included,
- Python
- SQL
THE IMPACT
With the new demand forecasting solution, the company’s business users were provided with weekly product level demand forecasting reports that had over 95% accuracy. This helped the company right size their manpower and fleet sizes, reduce inventory costs and existing wastage in the packaging, handling, and processing of orders. In turn, this also lowered the overall cost per drop metric for the company.
By pre-emptively getting the required stock from their suppliers, the company was able to ensure that over 85% of all products were available throughout the year. Prior to the implementation of this demand forecasting system, at least 50% of all their products were unavailable during the year.