Understanding the Power of Data Analytics in Today’s World
In today’s fast-paced digital landscape, the ability to harness the power of data has become a cornerstone of successful business strategy. Data analytics is not merely a technical tool; it is a transformative force that enables organizations to navigate the complexities of modern markets with precision and agility.
The significance of data analytics is underscored by its capacity to inform decision-making, optimize operations, and enhance customer experiences. Companies that effectively leverage data analytics are not only able to identify trends and patterns but also predict future outcomes, allowing them to stay ahead of competitors and respond swiftly to changing market demands.
The data analytics market size was valued at USD 41.05 billion in 2022 and is projected to grow from USD 51.55 billion in 2023 to USD 279.31 billion by 2030, exhibiting a CAGR of 27.3% during 2023-2030.
How Data Analytics has evolved over the years
Data analytics has undergone significant transformation over the years, evolving from simple manual processes to sophisticated, AI-driven methodologies. This evolution can be categorized into several key areas, each marked by technological advancements and changing business needs.
Early Beginnings
Data analytics originated in the 1950s, when companies began manually collecting data to improve operations. Early analytics roles were technical, focused on managing data rather than generating insights.
The Spreadsheet Era
In the late 20th century, Microsoft Excel popularized data analysis, enabling anyone—not just experts—to handle tasks like financial modeling and budgeting, with structured, easy-to-manipulate data.
The Rise of Big Data
By the 1990s and early 2000s, the internet and connected devices sparked the big data era, driving organizations to adopt advanced databases and analytics tools to manage vast data volumes and extract valuable insights.
Business Intelligence and Cloud Computing
As big data matured, so did the need for more sophisticated analytics tools. The emergence of Business Intelligence (BI) platforms in the early 2000s allowed non-technical users to create interactive dashboards and reports without extensive coding knowledge.
Current Landscape and Trends of Data Analytics
Today, we are in the AI-driven analytics era, where artificial intelligence and machine learning play pivotal roles in extracting insights from data. Modern analytics tools leverage advanced algorithms to predict trends and automate decision-making processes, significantly impacting various sectors including healthcare, finance, and retail. Some of the key trends are shaping the future of Data Analytics, let’s explore some of them.
AI-Powered Data Analytics
AI in data analytics is revolutionizing business by automating insights, boosting decision speed, and enhancing accuracy. Machine learning detects data anomalies and correlations, raising productivity by 50%, with 80% of employees reporting improved efficiency. The global AI market is projected to grow 37% by 2030.
Edge Computing
Edge computing processes data closer to its source rather than relying on centralized data centers. This trend is crucial for real-time analytics, reducing latency, and improving efficiency. The global edge computing market was valued at approximately $332.01 billion in 2023 and is projected to reach $432.94 billion in 2024, with an expected compound annual growth rate (CAGR) of 28% from 2024 to 2034.
Augmented Analytics
Augmented analytics combines traditional analytics with advanced technologies like natural language processing (NLP) and machine learning to automate insights generation. This trend democratizes data access, enabling non-technical users to engage with complex datasets intuitively.
Data-as-a-Service (DaaS)
The DaaS model will gain traction, providing organizations with on-demand access to high-quality data via the cloud. This approach simplifies data management and enhances agility, enabling faster decision-making.
Data Fabric
The concept of a metadata-driven data fabric unifies disparate data sources into a single layer that simplifies access and analysis. By leveraging metadata, organizations can manage their data more effectively, ensuring seamless integration and governance across various platforms. This approach is becoming increasingly vital as organizations grapple with vast amounts of fragmented data.
Predictive Analytics and Beyond
Data analytics encompasses a range of techniques used to analyze raw data with the aim of drawing meaningful insights. It plays a crucial role in decision-making across various sectors by transforming data into actionable information. Among the various branches of data analytics, predictive analytics stands out as a powerful tool that utilizes historical data, statistical algorithms, and machine learning techniques to forecast future outcomes.
Emergence of Predictive and Prescriptive Analytics
Predictive analytics has emerged as a vital component in the realm of data analytics, particularly as organizations seek to harness large volumes of data generated in today’s digital landscape. It builds on descriptive analytics— which summarizes past events— by not only explaining what happened but also predicting what is likely to occur in the future. This evolution has paved the way for prescriptive analytics, which goes a step further by recommending actions based on predictive insights.
Leveraging Data for Predictive Insights
Organizations are increasingly using predictive analytics to forecast trends and make proactive decisions. By analyzing historical data, they can identify patterns to drive strategy. Key applications include:
- Customer Behavior Prediction: Retailers analyze purchasing patterns to tailor marketing and optimize inventory.
- Risk Management: Financial institutions assess risks and detect fraud by analyzing behavior and transaction data.
- Operational Efficiency: Manufacturers use predictive maintenance to prevent equipment failures, reducing downtime.
Moving towards Cognitive Analytics
Cognitive analytics is an advanced form of data analysis that combines artificial intelligence (AI), machine learning (ML), and natural language processing (NLP) to process and analyze large volumes of structured and unstructured data. This technology simulates human thought processes to extract insights, recognize patterns, and make informed decisions, thereby enhancing traditional data analytics approaches.
Understanding Cognitive Analytics
Cognitive analytics operates by mimicking human cognition to interpret complex datasets. It integrates various analytical techniques to provide context-aware insights, which are particularly useful in environments where data is often unstructured, such as social media interactions, customer feedback, and multimedia content.
Key Features of Cognitive Analytics:
- Human-like Intelligence: It processes information similarly to the human brain, understanding context and meaning rather than merely performing calculations.
- Integration of Diverse Data Sources: It can analyze data from various formats including text, audio, video, and images to provide comprehensive insights.
- Real-time Decision Making: Cognitive analytics supports businesses in making timely decisions by analyzing current data trends and patterns.
Skills in Demand for the Data Analytics Workforce
As organizations increasingly rely on data-driven decision-making, the demand for skilled data analytics professionals continues to rise. Key skills that are currently sought after in this field include:
- Artificial Intelligence (AI): Proficiency in AI is essential as it enhances data analysis capabilities, allowing for more sophisticated insights and predictions.
- Machine Learning: Understanding machine learning algorithms is crucial for developing predictive models and automating data processes.
- Data Governance: Knowledge of data governance frameworks ensures compliance with regulations and ethical standards, which is increasingly important as data privacy concerns grow.
- Data Visualization: Skills in tools like Tableau or Power BI are vital for presenting complex data insights in an understandable format.
- Statistical Analysis: A strong foundation in statistics is necessary for interpreting data accurately and making informed decisions.
Prescience Insights
A major E-commerce firm aimed to boost revenue and profits by refining its ineffective pricing strategy. By leveraging data analytics to identify optimal price points and categorizing SKUs into modules, the team utilized algorithms like Recurrent Neural Networks for price optimization. As a result, revenue increased by 8% and profit margins improved by 1.5% within six months, while the client’s competitive daily pricing capability rose by 60%.
Click here to get a detailed information
Prescience Data Solutions showcases the expertise in elevating data capabilities through maturity assessments, offering descriptive, predictive, and prescriptive analytics. It includes services like data visualization and solutions for common challenges like data quality and integration. Positioned as a strategic partner, Prescience helps organizations optimize data usage with expert consulting and tailored strategies.
Final Takes
In conclusion, the significance of data analytics in today’s business environment cannot be overstated. It is a transformative force that empowers organizations to leverage data for strategic advantage, driving efficiency and enhancing customer experiences. As technology continues to evolve, the future of data analytics will likely bring even more innovative solutions that further integrate AI and machine learning into everyday business practices.
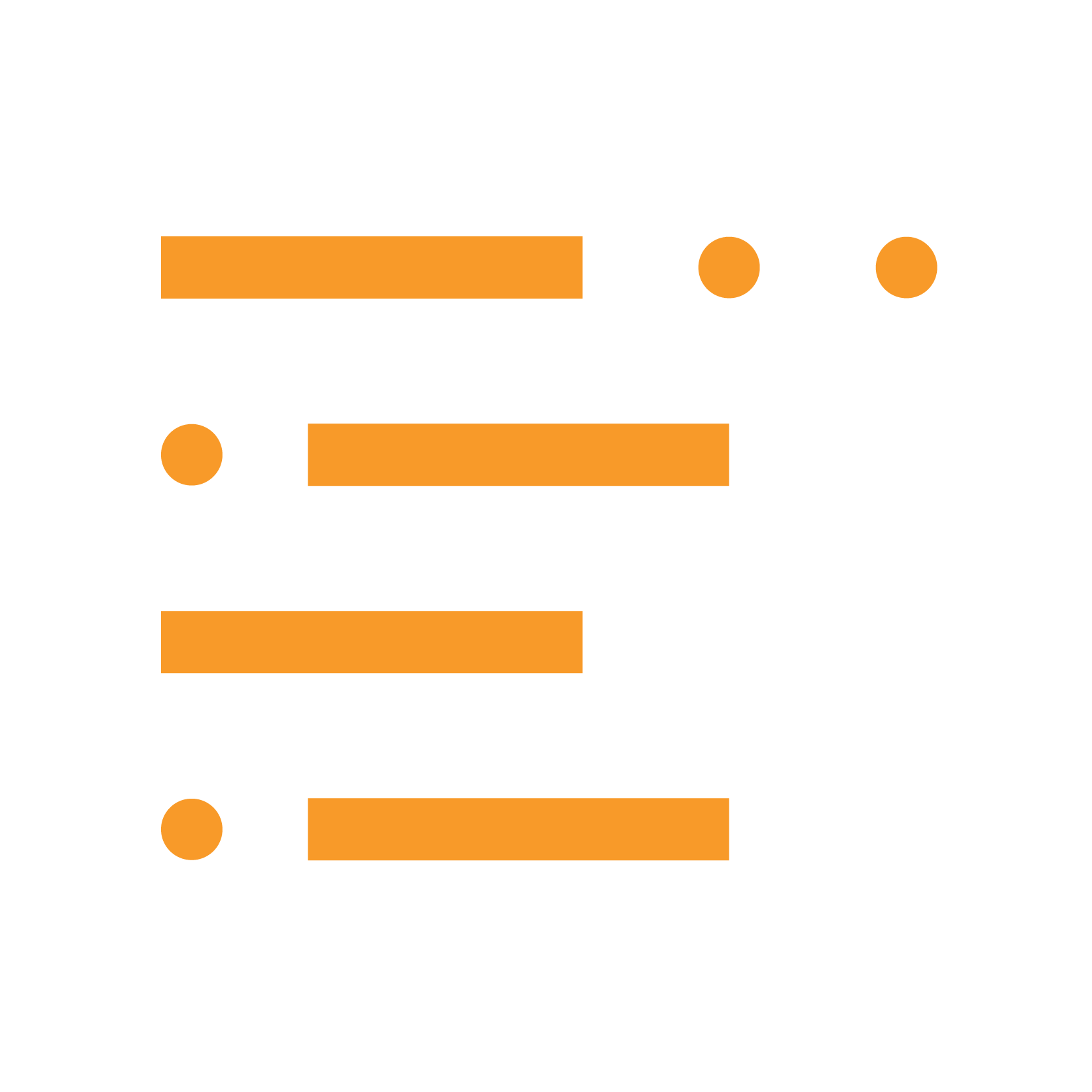
Prescience Team