Table of Contents
2. Why MLOps Matters in Logistics and Supply Chain
3. Key MLOps Strategies for Transformation
- Demand Forecasting and Inventory Management
- Predictive Maintenance of Fleet and Equipment
- Route Optimization
- Enhancing Customer Experience
The term MLOps was first coined in a 2015 research paper called, “hidden technical debt in machine learning system”. This paper outlined the challenges of dealing with large volume of data sets and how using Devops will help in managing ML practice better.
MLOps stands for machine learning operations. It is a method that controls the lifecycle of a machine learning model. It combines both machine learning and DevOps (a combination of software and operations to maintain the lifecycle of software). For large-scale productions, MLOps not just help streamlining but also help in the deployment of ML models. Moreover, integrating MLOps will help reduce constant friction between data scientists and the tech operators, as the models can automate the routine tasks by themselves.
MLops helps businesses to build ML models and deploy them at scale for various internal needs.Traditional machine learning (ML) solutions often remained stuck at the pilot stage and failed to scale into real-world applications. MLOps (Machine Learning Operations) addresses this challenge by enabling seamless integration of ML models into production environments.
Unlike standalone ML solutions, MLOps offers a robust pipeline that automates the entire workflow—from data ingestion and model training to deployment and monitoring logistics and supply chain, implementing MLOps strategies is of utmost important to get real-time insights, demand forecasting and route optimization etc.
In this blog we will explore four important MLOps strategies that helps transform logistics and supply chain management.
- Demand forecasting and inventory management
MLOps helps in demand forecasting by deploying ML models. ML models analyze sales trends, regional demand. Intelligent Audit systems monitor model predictions in real-time, flagging deviations and triggering updates. Traditionally, demand forecasting relied heavily on static models or manual estimation. Today businesses leverage MLOps to deploy scalable, retrainable demand forecasting models with the help of Intelligent Audit systems.FMCG (fast moving goods) uses MLOps for demand forecasting which helps reduce stockouts, reduces inventory costs and improves accuracy etc. - Predictive maintenance of fleet and equipment
Predictive maintenance of fleet and equipments means to use ML models to predict the equipment failure beforehand. ML models analyze the past patterns in equipment failure and identify anomalies It uses real-time data to do this process. MLOps helps in continuous automation of model updates as new data gets added in. This continuous ML models to get accurate in prediction over a period. Additionally, MLOps ensures these models are continuously refined to identify and mitigate risks promptly. - Route Optimization
ML models are used to optimize delivery routes. This helps in maintaining the delivery of goods in a smooth way. This helps analyze traffic, weather, and delivery schedules for finding the most efficient routes, thus reducing delays, fuel consumption, and delivery times. MLOps helps in content retraining of the models with new data, and deployment, which helps keep predictions accurate with the changing conditions. - Enhancing Customer Experience
Machine learning models are used to Improve customer experience with personalization. These models are trained to evaluate customer preferences, behavior patterns, and purchasing history. Recognizing the needs of the individual customer it allows businesses to create personalized recommendations and targeted content throughout the digital touchpoints. MLOps serve a key component for the operationalization of these models so they can be continuously integrated and monitored for retraining as new customer data is added to the models.
Real-life example
A Fortune 500 company, a major player in transportation, supply chain management, and logistics, which operates seven hundred container vessels across 130 countries faced challenges in the third-party logistics department (outsourcing to an external provider). The challenges faced includes warehouse restrictions, unexpected spikes in demand, increasing costs, talent shortages, etc.
With the motive of staying ahead in the competition, the company invested in new technology, especially ML models. This led the company to build different ML models catering to the specific needs of each department. However, this led to a proliferation of 1300 ML models built on various platforms, making it difficult for the company to handle the expenses.
To solve this problem, the company partnered with Prescience Decision Solutions to create an enterprise-level MLOps (machine learning operations) platform. The team assessed the existing ML models and set up new principles for building the MLOps platform, such as simplicity, scalability, security, & reliability.
The team builds an MLOps platform using tools like Redis, PostgreSQL, Kubeflow, Git, MLFlow and Azure Kubernetes Services. They successfully migrated four existing ML models to the new MLOps platform. MLOps significantly improved demand forecasting by centralizing model tracking, enabling seamless lifecycle management and automated monitoring to maintain accuracy. Auto-scaling ensured high performance during demand surges, while standardized workflows reduced operational costs.
To get more insights, click here.
Conclusion
MLOps is transforming logistics and supply chain by making ML models easier to deploy, scale, and manage. It turns pilot projects into real solutions with real-time insights, automation, and continuous updates. MLOps helps in enhancing customer experience to demand forecasting by maintaining the ML models lifecycle.
At Prescience Decision Solutions, we offer complete data solutions that integrate artificial intelligence and machine learning across various services like analytics, business intelligence, data engineering, and more.
Explore our customers success stories here.
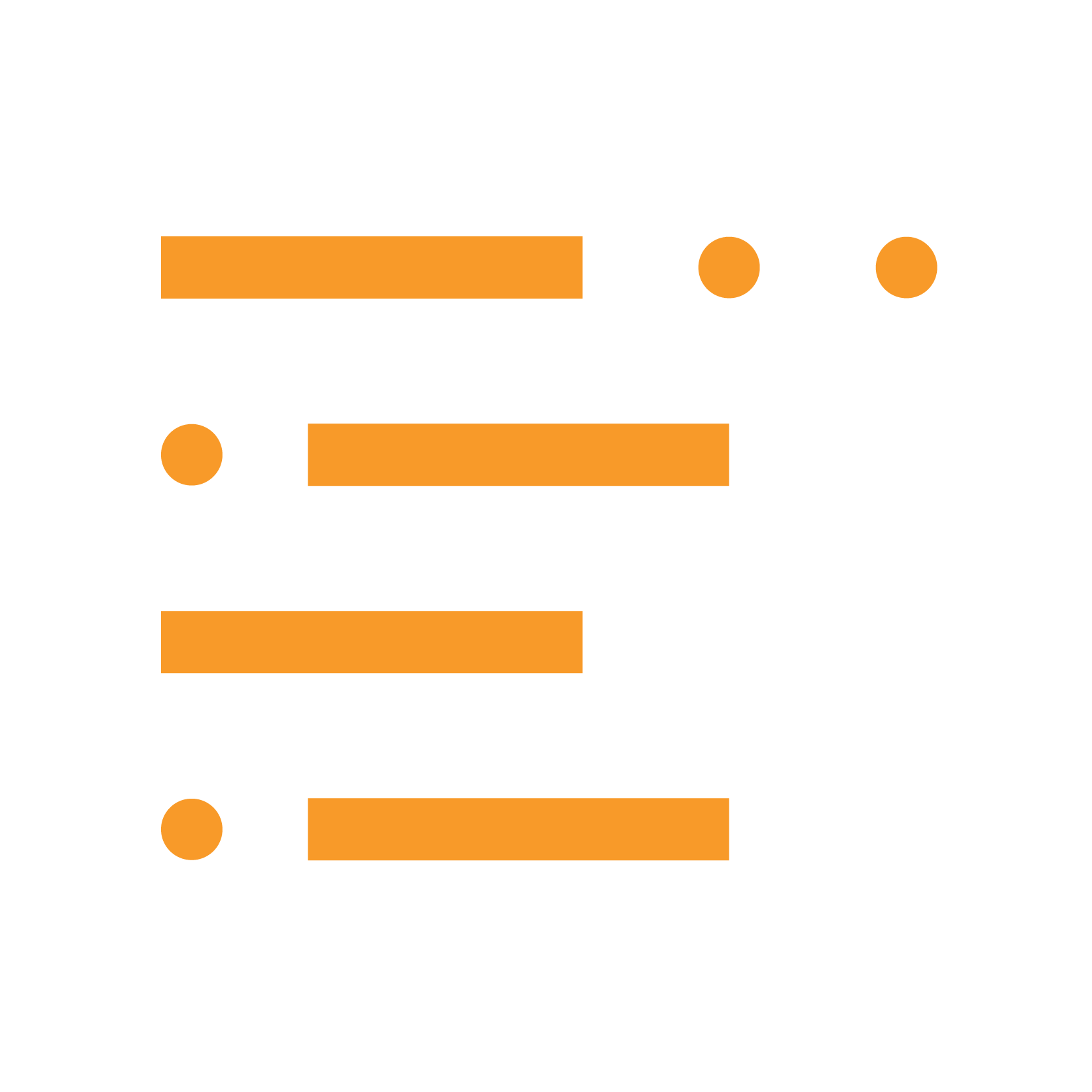
Prescience Team