Table of Contents
- The Growing Complexities in Supply Chain Management
- The Role of Data Analytics in Transforming Logistics
2. Key Applications of Data Analytics in SCM
- Demand Forecasting
- Route Optimization
- Warehouse Management and Optimization
- Supplier Performance Monitoring
- Risk Management
- Case Study: Enhancing Supply Chain Efficiency with Data Analytics
- The Future of Data-Driven Supply Chain Management
- How Prescience Decision Solutions Can Help
Logistics and supply chain management represent one of the most critical aspects of the world economy. These functions provide services like logistics, connecting sellers with buyers, and driving economic growth.
In the modern interconnected marketplace. supply chain management faces an array of complex challenges. From order processing, managing inventories, to improving the overall operational efficiency of the businesses, businesses are facing rising complexities that are hard to tackle using traditional methods.
The field of data analytics is transforming the logistics and supply chain management functions. The traditional practices once static and based on long-term predictions is now replaced by real-time data analysis, predictive modeling, and automation.
In this blog, we will explore five different ways data analytics is transforming supply chain management and logistics.
- Demand Forecasting
Demand forecasting is an important component of supply chain and logistics operations. It enables businesses to anticipate customers’ needs and adjust the inventories accordingly. It also helps in efficient stock management and avoiding the costly mistakes of overstocking or understocking.Predictive analytics play a significant role, by analyzing historical data and considering external factors seasonality and market trends. Additionally real-time analytics provides live sales data, current social media trends, weather changes, and other variables that impact demand patterns. - Route Optimization
Route optimization is identifying the most efficient, and cost-effective, delivery routes considering factors such as traffic, delivery time frames, and vehicle capacity. The logistics department uses real-time data to determine the most efficient routes, analyzing factors like traffic patterns, weather conditions, and delivery constraints. Additionally, AI and ML algorithms analyze the data to select optimum routes. - Warehouse management and optimization
Advanced analytics plays a significant role in managing warehouse operations. AI and machine learning algorithms enables real-time data analytics to optimize space utilization, and inventory management. Automated inventory tracking is done through RFID, IoT sensors, and computer vision which reduces manual checks and ensures real-time stock visibility. Moreover, from route optimization to stock management, predictive analytics plays a crucial role in improving operations efficiency and making warehouse management easier. - Supplier performance monitoring
Big data in supply chain management is an important tool that for understanding supplier behavior in real-time. Businesses can use data to determine important aspects like lead times, defect rates, and compliance, which allows them to be data-informed when selecting and managing suppliers.By analyzing these aspects, businesses can pinpoint the trustworthy partner, build strong relationships with the supplier, and take proactive care of the potential consequences. This, in turn, helps mitigate the delays and problems with product delivery, thus making the management process smooth. - Risk Management
Risk management is vital for successful navigation of business uncertainties and the maintenance of operational stability. Predictive analytics helps in exposing potential risks by monitoring the impacts of the geopolitical events, weather, and the market fluctuations.Businesses can plan for uncertainties beforehand by analyzing historical and real-time data. This proactive approach not only make supply chain perform better but also decrease the delay, financial losses, and reputational damage. Businesses that integrate predictive analytics in their operations can implement data-driven solutions, making supply chain operations more flexible and resilient to unforeseen obstacles.
Real World Example in Logistics and Supply Chain Management
A global e-commerce platform, serving 2 million customers faced inefficiencies in its supply chain, manpower, and logistics, leading to frequent stockouts and high costs. By incorporating data analytics, the team at Prescience Decision Solutions implemented an analytics-based demand forecasting system using historical and third-party data.
The team used fundamental ML methods like linear regression, random forest, XGBoost, and VAR. Through this, the business achieved 95% accuracy in product-level demand forecasts, reducing constant stockouts, optimized inventory, fleet management, and reducing overall expenses. Moreover, this approach improved product availability from 50% to 85% year-around.
To get a detailed insight click here.
Conclusion
Supply chain management and logistics are transformed by data analytics assisting businesses to navigate complexities seamlessly with utmost efficiency. Data-driven insights enhance demand forecasting, route optimization, warehouse management, and risk mitigation. This empowers businesses to make informed decisions, cost reduction, and improve operational resilience.
By leveraging advanced analytics, AI, and machine learning, businesses can streamline their supply chain operations and stay competitive.
At Prescience Decision Solutions, we offer complete data solutions that integrate both Artificial intelligence and Machine learning across various services like analytics, business intelligence, data engineering and more.
Moreover, we offer comprehensive supply chain and logistics analytics services designed to boost efficiency of operations across the entire value chain —- from procurement and manufacturing to transportation and logistics.
Discover successful customer stories here.
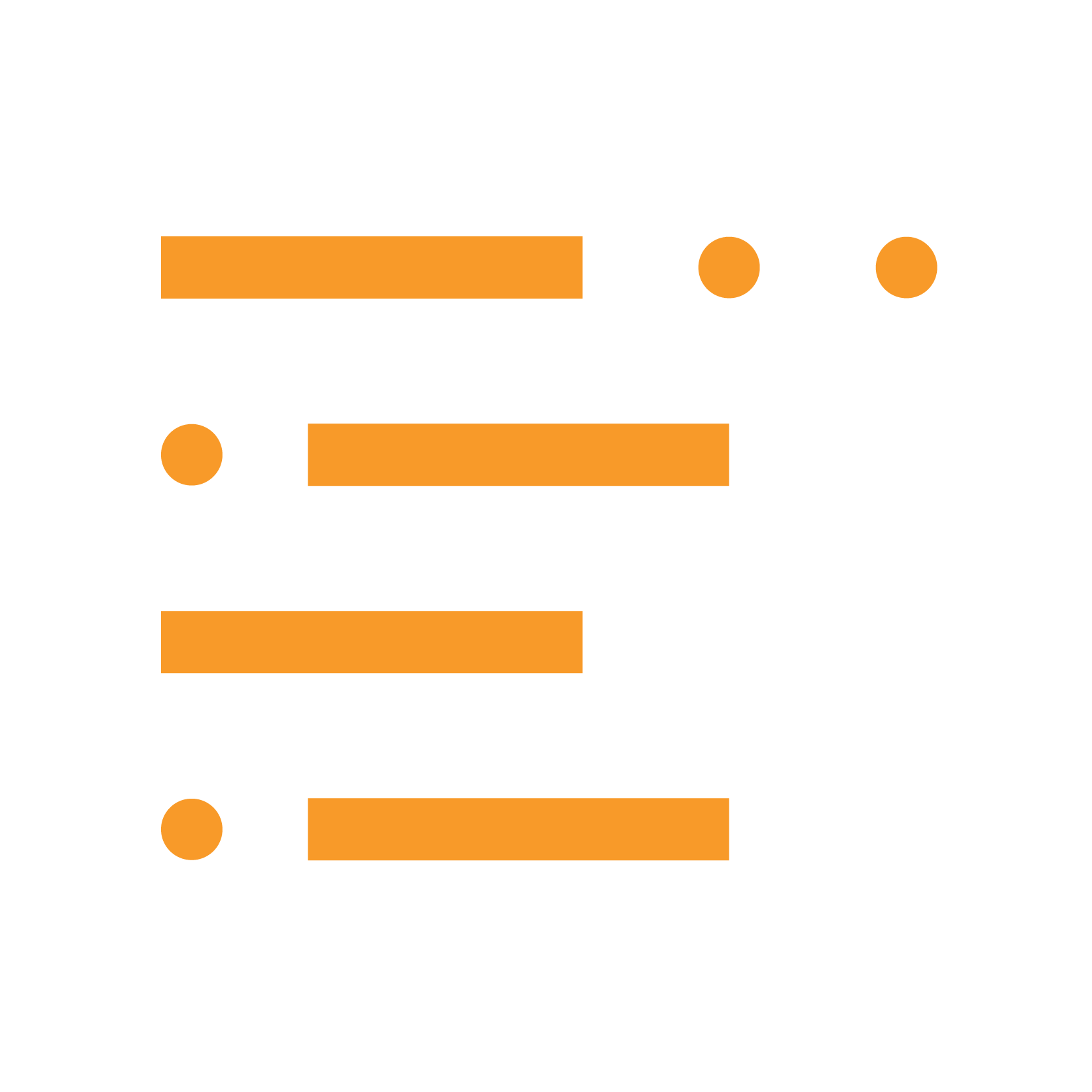
Prescience Team