Understanding personalization in e-commerce
With the rapid growth of e-commerce, personalization has become a necessity. Customers are looking for more than just a mere transaction; they want the brands to understand and acknowledge their preferences. Aware of this shift, businesses are constantly trying to meet these expectations by offering personalized products and experiences.
Personalization simply means a practice of creating personal interactions and experiences addressing the specific needs of customers online. Personalization can only happen when the right dataset is available for analysis, providing the insights needed to understand customer preferences. Thus, making data a foundation for creating personalized solutions for customers.
In this blog, we will explore the importance of data in personalization, its applications, key benefits, and how to measure personalization success through various metrics.
Importance of Data in Personalization
Data plays a pivotal role in personalization, helping businesses to understand and cater to the specific needs of individual customers. This involves collecting data based on various factors, such as demographic data, transactional data, behavioral data, and so on.
According to research, 83% of consumers are willing to share their data for personalized experiences, indicating their strong desire for customization in their shopping journeys.
By leveraging advanced analytics—such as predictive and real-time analytics—along with machine learning algorithms, businesses can gain insights into customer behavior and preferences.
Let’s explore the different types of data helping in providing personalized products and why data quality is key to accurate insights.
Types of Data for Personalization
Demographic Data: Demographic data includes personal information (age, gender, income level, location, and other characteristics that help segment the audience). This is often done through surveys, polls, and focus groups (small groups of people brought together to talk about a product or service). Businesses utilize these discussions to understand customers’ likes and dislikes.
Transactional Data: Transactional data refers to the records of past purchases made by customers. Online shopping platforms track all transactions made by users. Analyzing this purchase history helps identify buying patterns and preferences of various customers, which helps in preferred product recommendations increase the likelihood of repeat sales.
Behavioral Data: Behavioural data refers to the overall interaction made by the customer on the digital platform. This includes websites visited, time spent, browsing history, etc. Behavioural data is collected through web analytical tools that track user interaction on the site. Additionally, through CRM systems, that can collect detailed customer records over time.
Social media interactions: This includes engagement that takes place on social media sites, like personal shares, comments, and other forms of engagement. Social media analytics tools(software applications) can track user interactions in real time, allowing brands to understand which content resonates most with their audience. Thus, businesses can leverage this data to create content that resonates with their audience.
Why data quality matters
The success of personalization strategies is heavily dependent on the quality of the data collected. If the data collected from various sources does not meet the key data quality criteria, such as accuracy, relevance, timeliness, comprehensiveness, and consistency, then it might lead to poor decision-making, wrong customer insights, etc.
Following are the key reasons why quality data is essential:
Informed decision-making: Quality data allows businesses to make decisions based on factual insights rather than assumptions.
Enhanced Customer Insights: Accurate data provides deeper insights into customer behavior and preferences when compared to poor data quality. This helps brands create more relevant content aligning with customer needs.
Improved Segmentation: High-quality data facilitates more granular segmentation (demographic, behavioral, psychographic) of customer bases, thus helping brands to offer personalized products catering to customer needs.
Key Applications of Data-driven Personalization (Role)
Customer segmentation
Leveraging data analytics enables businesses to categorize customers into distinct segments based on factors like demographics, purchasing history, and online behavior. By analyzing these datasets, e-commerce platforms can identify groups like frequent buyers, occasional shoppers, or those who respond to specific promotions. This segmentation helps businesses create tailored marketing campaigns, pricing strategies, preferred product development, etc. focusing on each segmented group, enhancing customer engagement and conversion rates.
Product Recommendations
Personalized recommendations of products can boost conversion rates by 288% and reduce cart abandonment by up to 4.35%. By analyzing transactional (past purchases) and behavioral data (browsing behavior), e-commerce platforms can suggest products that align with customer tastes and preferences.
For instance, if a customer frequently buys gadgets like smartphones or headphones, suggesting complementary items such as phone cases and screen protectors will enhance the shopping experience of the customer.
Price Optimization
Data analytics plays a major role in setting dynamic pricing strategies for various customers. This is based on data collected from customer purchase history, market data, transaction data, demographics, etc. Product prices are set, or personalized discounts are offered to specific customer segments based on data-driven reports.
Additionally, real-time analytics is used for real-time price adjustments based on the current market conditions, customer behavior, or other influencing factors. This approach leverages live data and advanced algorithms to ensure pricing remains competitive, profitable, and aligned with customer demand.
Dynamic Content personalization
Brands can customize their content, including logos and information, to align with customers’ preferences and past interactions on their website or app. By leveraging data analytics, businesses can understand user interests based on browsing history and behavioral patterns. Tracking customer behavioral data (customer engagement on site) enables more accurate product recommendations and personalized user experiences.
For instance, if a user shows interest in fashionable products, the site can display banners, blog posts, or product descriptions more towards fashion. This enhances the shopping experience, making it more engaging, relevant, and satisfying for the user.
Key benefits of data-driven personalization
Implementing data-driven personalization offers numerous advantages
Enhanced Customer Loyalty: Personalized experiences encourage more repeat purchases and build brand loyalty. Customers are more likely to return to brands that understand their needs and acknowledge them.
Competitive Advantage: Brands that leverage personalization effectively can differentiate themselves from competitors, making it more likely that customers will choose them over other brands.
Higher Customer Lifetime Value: Personalized interactions can increase the lifetime value of customers by encouraging ongoing engagement and repeat business.
Higher ROI on Marketing: Personalization optimizes resource use by targeting strategies that resonate with specific customers, boosting the effectiveness of marketing campaigns.
Key metrics for measuring personalization Success
Customer-Centric Objectives: Instead of only tracking metrics that benefit the company (like sales or profits), it is important to focus on measuring metrics that value customers. This includes tracking whether customers find product recommendations relevant, measuring how much time customers save using your service, and collecting customer satisfaction scores or feedback. By focusing on these metrics, businesses can better understand the impact of personalization efforts in enhancing the customer experience.
Time Frame Considerations: Consider utilizing short-term metrics like (e.g., click-through rates) for immediate feedback and medium-term metrics (e.g., customer journey engagement) to assess ongoing interactions and long-term metrics (e.g., customer lifetime value) to evaluate overall relationship impact.
Engagement Metrics: Track user engagement through click-through rates, time spent on pages, bounce rates, and social media interactions. These metrics provide insights into how well personalized content resonates with users and how often they are likely to visit the website.
Qualitative Feedback: Collect qualitative data through customer surveys and feedback mechanisms to gain insights into customer perceptions of personalized experiences. This can complement quantitative data for a more rounded evaluation
Conversion Metrics: This means tracking how well a business’s sales process is working by looking at how many people move through each step (funnel progression) and conversion rates (how many end up making a purchase). This helps businesses to assess whether their personalized marketing or sales strategies are boosting sales.
Key indicators include the average amount of money spent per order (average order value) and how much money your business is making overall (revenue growth), which show whether your business is financially successful.
Final Take
With the above discussion, we reach the conclusion that personalization is crucial for the success of e-commerce platforms. It provides a progressive approach to meet consumer expectations and deliver customized experiences. By harnessing high-quality data from various sources, businesses can understand and cater to individual preferences, significantly improving customer satisfaction, loyalty, and engagement.
This helps businesses to increase sales, higher conversion rates and higher customer lifetime value, empowering them to succeed in an increasingly competitive landscape. Today, businesses embracing personalization methods will build meaningful futures with their customers, ensuring long-term growth.
Prescience Decision Solutions plays a vital role in enabling this personalization. While many e-commerce platforms provide generic, off-the-shelf capabilities, Prescience offers something unique—custom AI and data science solutions tailored to the specific needs of businesses. By addressing unique use cases, Prescience helps organizations deliver truly personalized experiences, setting them apart in the marketplace.
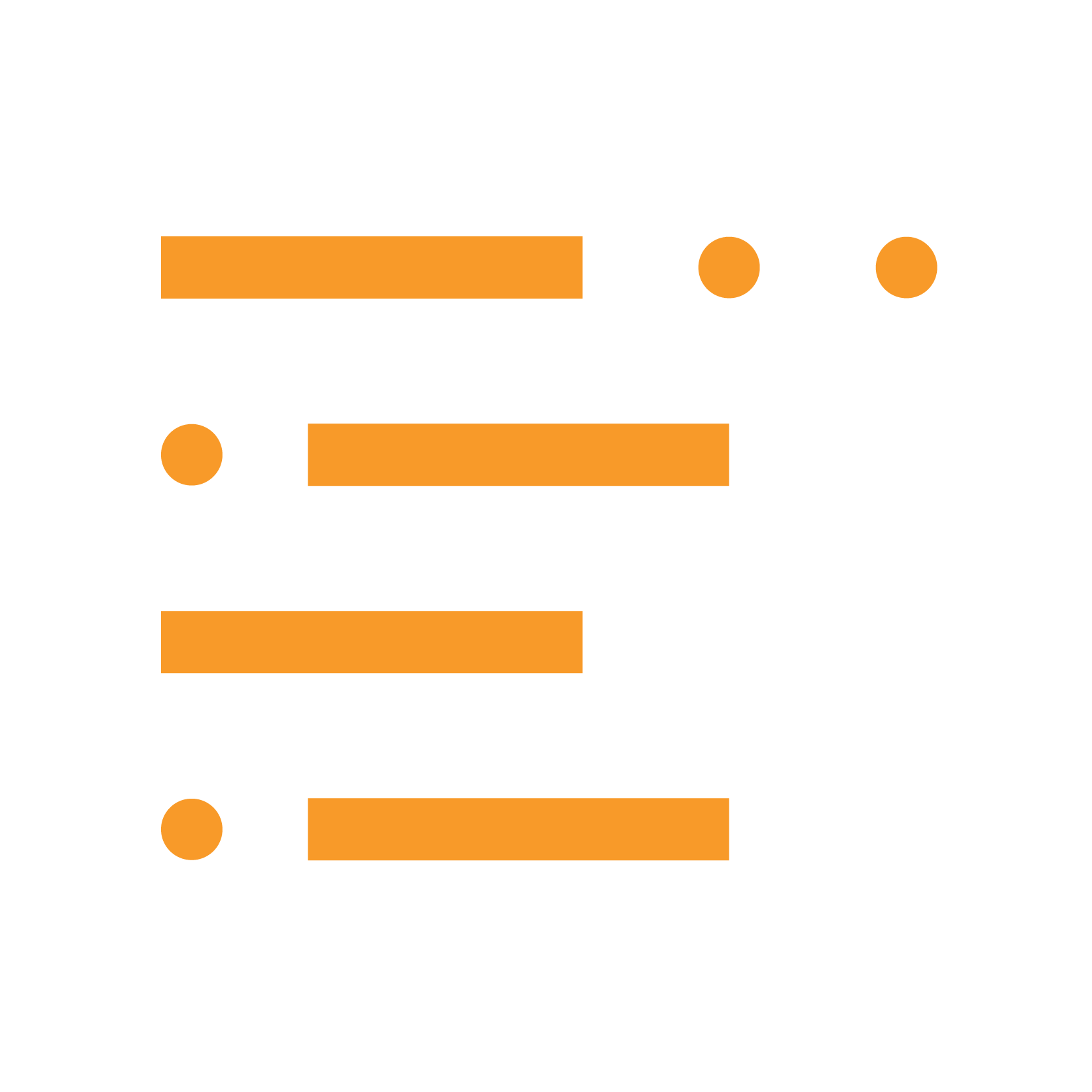
Prescience Team