While investing in AI, enterprises need be prepared for multiple dimensions to truly embrace the power of these technologies and keep pace with the rate of change. An AI maturity framework provides a comprehensive evaluation of an organization’s readiness, assessing its capability to successfully integrate AI.
In absence of a readiness evaluation, enterprises risk failures due to poor planning and execution gaps. While artificial intelligence is seen as one of the greatest opportunities of our time, many organizations face various challenges in its adoption, limiting AI’s potential to unfold at its optimum level. An AI maturity assessment can offer good insights into your current position and a roadmap to move forward with confidence.
Identifying your capabilities and adoption levels should be the first important step toward success. It involves evaluating readiness across various dimensions like overall strategy, budgeting, data, infrastructure, etc. Here are some key considerations, not all of which require to be at the same maturity level for an organization to start their AI journey:
Vision and Strategy:
A clear vision and strategy are the foremost requirements for successful integration of AI into overall business strategy and roadmap. Along with this, allocation of budget to various AI initiatives or integration of this budget with the business initiative are crucial aspects to look at while evaluating AI maturity at organizational level.
- Integration of AI into overall strategy: Challenges are different for organizations at different stages of AI integration. The highest level of maturity here is integrating “AI seamlessly into overall strategy of the organization”. At initial stages organizations are mostly exploring the potential while it is completely different for another company that already has AI as one of the components of business strategy.
- Budgeting of AI projects: Budgeting takes places across stages, for example a part of it is allocated for testing ideas during Proof of Concepts (PoCs). This is the initial budget, which mostly covers cost of data, software, and AI consulting team, etc, and is also useful in identifying and addressing potential risks and failures. Corresponding budgets come into the play at later stages when any AI project evolves into much advanced levels. Whatever be the stage, proper allocation of budget for each phase including, PoC, pilot project as well as full scale implementations is a defining metric to gauge the success of any AI project in advance. This can help manage resources effectively and safeguards an organization’s commitment towards the AI implementation journey.
Business integration and use cases
Evaluating the alignment of existing business practices and policies with the potential impact of AI adoption is crucial. This includes understanding how AI can be integrated into existing workflows and processes without disrupting current business operations.
- AI adoption across the organization: There are various stages from initial awareness to full integration and can range from “Just learning about AI” to “Integrating AI across various business workflows”. It usually starts from educating the team about potential benefits, followed by experimentation and pilots to test certain AI capabilities in specific business functions. At a higher maturity level AI is integrated across business workflows and ultimately acts as a core component of the enterprise, driving continuous improvements and supporting strategic decision making.
- Methodology for AI use case development: At the highest level of maturity there is a standard procedure to profile and detail all AI use cases consistently. Often, organizations lack clarity on how AI can enhance processes or solve business problems. While use cases can be identified based on immediate requirements or specific projects, a more structured approach involves cross-functional teams assessing the potential impact of AI. Thoroughly analyzing each potential use case and evaluating factors such as feasibility, resources, and alignment with goals should be a standardized procedure.
Read more: The Enterprise Playbook for Generative AI Implementation and Adoption
Data
Availability and quality of data lay the foundation for successful AI adoption. An organization’s access to high-quality data from multiple sources in large volumes largely simplifies their AI journey. While assessing data-related aspects of AI maturity, the goal is to understand how an organization manages data accessibility and quality.
- Access to data while experimenting with AI: Low maturity organizations often have Challenges in accessing data while organizations at the higher end of maturity have ‘Data accessible across the entire entity through an integrated platform is the highest level of maturity’. Scattered data, incompatible data, or lack of data are common limitations companies may face in the initial stages. To conduct relevant AI experiments, standardized data formats and basic data integration projects are needed early on. At a more advanced stage, data integration from different sources involves centralized data storage solutions, such as data warehouses or data lakes, which ensure easy access. Data accessible across the entire entity through an integrated platform is the highest level of maturity.
- Data Quality: This is one of the most critical components of AI readiness, as the reliability and performance of AI models are directly correlated with the quality of data. An active data quality initiative with automated infrastructure and tools is the highest maturity level that enables organizations to utilize data assets most effectively (check out Precience Data Sentinel, a Data Quality solution available on AWS Marketplace and Microsoft Azure Marketplace). Without well-defined processes, organizations cannot assess, manage, and improve data quality leading to the lowest maturity level. Some organizations may have basic data cleaning and validation processes in place, but these may not be adequate for large-scale AI implementations. Having active data quality initiatives with automated infrastructure and tools is the highest level of maturity and ensures real time data quality monitoring, which in turn, helps data scientists build reliable and accurate AI models.
IT Infrastructure
Assessing IT infrastructure is critical to the AI maturity framework as it significantly impacts the efficiency and scalability of AI initiatives. Adopting the right infrastructure suitable for running AI workloads ensures a supportive technological foundation, which is a primary requisite for AI development and deployment.
- Platforms and tools for deploying AI: A ‘Scalable and governed AI platform integrated across the organization to deploy AI models and streamline data access from ingestion to consumption’ is sign of an organization that is able to utilize AI most effectively. At an initial stage, there may be a lack of tools and platforms required to deploy AI, this is the lowest stage of maturity in an organization. Some are at a stage where they have access to basic AI tools and platforms, like subscription based or cloud-based services, but this too is not sufficient for large scale AI initiatives. The highest level of maturity is when an organization has a scalable and governed AI platform integrated across the organization to deploy AI models and streamline data access from ingestion to consumption.
- Computing infrastructure for AI development and operations: Digital natives and AI first organizations typically have an Optimized infrastructure provisioned for AI which can be scaled up / down as needed’. While organizations with basic infrastructure can support small AI projects, larger initiatives require high-performance computing clusters, high-capacity storage, and an optimized infrastructure. An ideal scenario involves having an optimized infrastructure specifically provisioned for AI, which can be scaled up or down as needed to support advanced AI development and operations seamlessly.
Read more: The Enterprise Playbook for Generative AI Implementation and Adoption
People
The availability of skilled talent to drive AI-related initiatives is crucial for driving successful AI adoption and creating long-term value. Every organization needs a structure to work with partners to get the right skill sets when needed. Clear roles ensure smooth collaboration across departments, which is a key consideration for seamless AI integration across the organization.
- Establishment of AI roles and responsibilities: AI initiatives are most effective when AI is treated as an integral part of organization, sponsored by top leadership and driven by AI champions. While at the beginning organizations either do not have any specific position that dedicatedly manages AI, or it’s taken care of as an additional responsibility by one of the team members. The role of dedicated AI teams is to align organizational goals with AI initiatives and integrate AI across functions by collaborating across departments.
- Methodology for developing AI knowledge and upskilling AI capabilities: A Concerted effort to develop AI literacy across the organization and align AI as a key component in the learning plan is needed to develop AI competency across the enterprise. While evaluating the people dimension of AI maturity, it’s important to review whether an organization is committed to fostering AI talent continuously. We need to consider if there are concerted efforts to develop AI literacy across the organization and align AI as a key component in the learning plan.
Governance
A strong governance framework is a necessity for becoming an AI-mature organization. The framework acts as a well-defined approach to govern AI initiatives and establish checkpoints before rollout. This is an ongoing framework that helps in understanding the challenges and opportunities associated with AI adoption.
- Degree of governance to enable AI: Many organizations at this stage have no or a make-do structure to govern AI. The lack of a dedicated AI governance body to review projects, set policies, and establish guidelines can result in potentially risky AI projects. Mature AI organizations have a well-defined and structured AI governance mechanism that has clear accountabilities across different stakeholders.
- Activities to ensure that AI is ethical and responsible: Ethical AI practices begin with educating teams about responsible AI. These practices include the development of ethical guidelines and conducting regular audits, which help address issues related to transparency, bias, and accountability. Integrating our AI ethics practices as part of the organization is the highest level of maturity that organizations should strive for.
Evaluation of AI use cases
While organizations can work on improving the maturity of processes across those various dimensions discussed, evaluation of AI use cases need to follow a structured value delivery framework. The value framework encompasses various aspects of the business. Below are some key points to consider:
- Business Functions and Impact: AI use cases should be evaluated based on their potential impact across different business functions. The need to assess the potential impact on various areas of the enterprise arises as the impact could vary based on the business process and maturity of the same.
- Collaboration and Improvement: Utilizing modular tools that facilitate collaboration across teams and improve models throughout the entire development life cycle can be a valuable aspect to consider when evaluating AI use cases.
- Risk: It’s crucial to consider the risks associated with AI use cases across different areas of the enterprise, including business lines, enterprise IT, customer service, compliance, and human capital. The risk could be related to cybersecurity, bias leading to poor implementation, safety risks, etc.
Read more: The Enterprise Playbook for Generative AI Implementation and Adoption
Other than these, several aspects must be considered while evaluating value generation from AI. The technology can be used effectively for a range of activities, from saving costs to improving long-term competitive advantage.
Cost Savings |
AI can reduce operational costs by automating repetitive tasks, optimizing resource allocation, and minimizing waste. Improved efficiency, reduced labor requirements, and streamlined processes collectively lead to overall cost savings. |
Revenue Growth |
AI can enhance revenue by identifying new business opportunities, improving customer experiences, and enabling personalized marketing. Predictive analytics and recommendation systems can drive sales and customer retention. |
Risk Reduction |
AI can mitigate risks by identifying anomalies, detecting fraud, and enhancing security. In finance, for instance, AI models can predict market fluctuations and manage investment risks. |
Quality Improvement |
AI can enhance product quality by identifying defects, optimizing manufacturing processes, and ensuring consistency. In healthcare, AI can aid in diagnostics and treatment planning. |
Customer Satisfaction |
AI-powered chatbots and virtual assistants improve customer support by providing quick responses and personalized assistance. Enhanced customer experiences lead to higher satisfaction and loyalty. |
Productivity improvements |
Automating tasks through AI reduces manual effort and accelerates processes. Time saved can be redirected toward more strategic activities. |
Innovation and Creativity |
AI can generate novel ideas, optimize designs, and assist in research. It enables organizations to explore uncharted territories and stay competitive. |
Strategic Insights |
AI-driven analytics provide actionable insights from large datasets. Organizations can make informed decisions based on data-driven recommendations. |
Employee Productivity |
AI tools can assist employees, improve collaboration, and enhance productivity. For example, AI-powered project management tools streamline workflows. |
Market Positioning |
Organizations that adopt AI strategically gain a competitive edge. AI can help position a company as innovative and forward-thinking. |
To ensure the relevant AI initiatives are prioritized, organizations should tailor their value measurement approach to their unique needs. The specific measures to be considered depends on the business context, industry, and the strategic goals of AI implementation. A customized approach can help gain even deeper understanding of the AI readiness process.
If you are looking for a starting point for your business, take advantage of our personalized FREE consultation workshop Sign up here.
Subscribe for regular updates on AI and Data Innovations, case studies, and blogs. Join our mailing list.
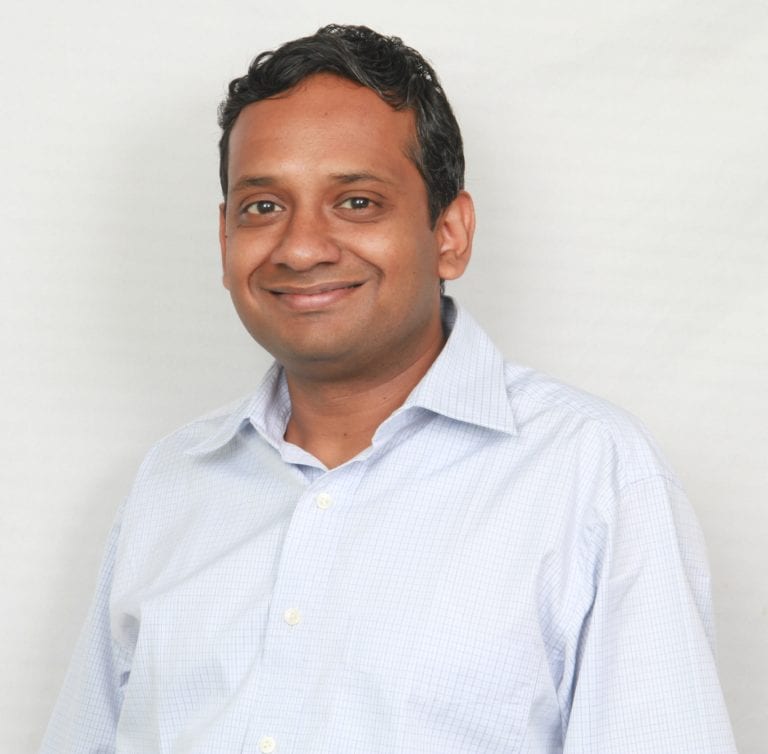
Shivakumar is a keen follower of scientific trends and an Asimov fan. He believes solid execution is the key to the success of any strategy and is focused on building a world-class data science team at Prescience. He has a B.Tech from IIT Delhi and an MBA from IIM Lucknow, with 20+ years of experience in the technology space.