Table of Contents
2. What is a Scalable Data Analytics Service, and Why Is It Important?
3. Key Features of Scalable Data Analytics Services
- On-Demand Scaling
- Seamless Integration
- Built-In AI/ML Capabilities
- Real-Time Data Processing
As enterprises grow, so does the amount of data they generate. Businesses must seek scalable data analytics services that can adapt in real time, integrate seamlessly with existing systems, and empower decision-making through AI-driven insights. Choosing the right analytics platform is not only about governing data; it is about establishing a foundation that scales with the business, creates optimal operational efficiency, and drives actionable intelligence.
In this blog, we will explain what scalable data analytics is and why it has become essential for modern enterprises.
What is a Scalable Data Analytics Service, and Why Is It Important?
A scalable data analytics service is a system that can efficiently manage increasing data volume and complexity without compromising performance. It is important for enterprises because it manages large datasets, ensures flexibility, reduces costs, and delivers real-time analysis. One of the main reasons enterprises adopt scalable data analytics services is that they allow analytical capabilities to keep pace with growth, eliminating the need for constant system upgrades.
Key Features of Scalable Data Analytics Services
- On-Demand Scaling:
With rising data volumes, enterprises need platforms that can scale resources instantly. This elasticity lets a system scale up or down as required. For example, a platform might need more processing power during peak hours when running large data files but less during off-hours. As businesses expand, data volumes grow; a scalable platform offers flexible, easy storage expansion. - Seamless Integration:
A strong analytics platform can pull and combine data from multiple sources—CRMs, ERPs, IoT devices, and third-party tools—into a unified view. This consolidated information enables businesses to make informed decisions quickly. - Built-In AI/ML Capabilities:
Modern analytics tools should do more than display data on dashboards; they should apply AI/ML to generate meaningful insights. Predictive ML models help identify future trends, detect anomalies, and drive personalization. Google Cloud Vertex AI, for instance, enables businesses to build and deploy ML models easily and integrates well with data analytics systems. - Real-Time Data Processing:
Real-time data processing examines information as it is created, enabling timely decisions and immediate action. Organizations rely on scalable data platforms to handle substantial data volumes and run real-time analytics efficiently. Stream-processing technologies such as Apache Kafka and Apache Flink continuously analyze data streams, delivering real-time insights.
Conclusion
Modern enterprises need scalable data analytics services that deliver real-time insights, seamless integration, and AI-driven decisions—while growing with the business.
At Prescience Decision Solutions, we navigate data science and analytics complexities across industries such as sales, finance, e-commerce, and marketing, offering comprehensive data analytics services that guide businesses from strategy formulation to technology implementation.
Explore our customer success stories.
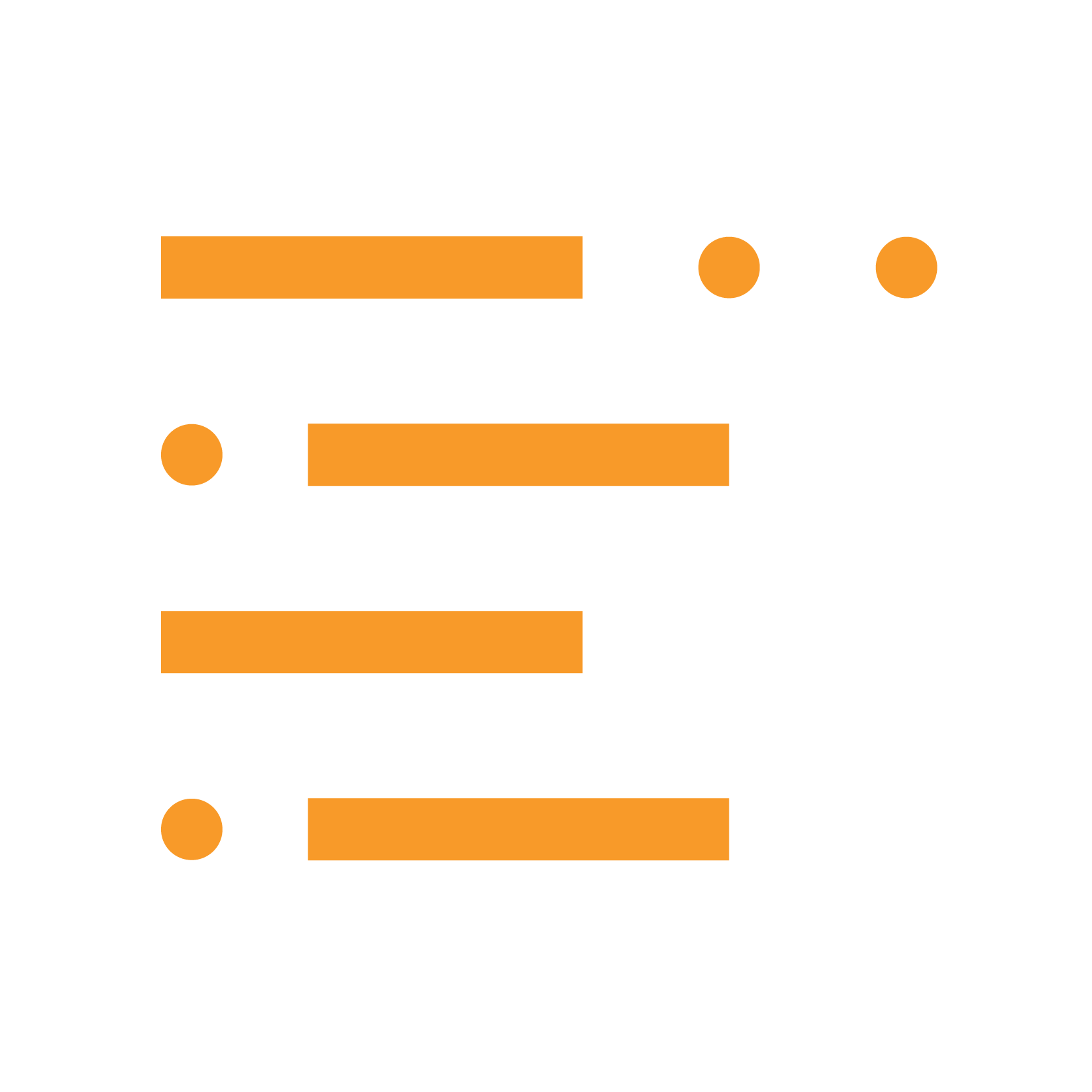
Prescience Team