Table of Contents
1. Introduction
3. Data Quality
8. Conclusion
1. Data Quality
One of the most important factors to consider while implementing AI is the quality of data. A data is said to be of good quality when it meets certain criteria’s such as accuracy, uniqueness, completeness, validity, timeliness etc. As organizations are growing their concern for data is growing equally, as it directly affects the company’s overall success.
A poor-quality data, such as incomplete data, duplicate data, missing values and so, creates negative impact on the business. According to Gartner report, it says every year due to poor quality of data it costs organizations $12.9 million, leading organizations in poor decision making.
Data quality can be improved and maintained throughout by following few measures like,
a. Set data governance policies—Data quality can be achieved by setting up policies like roles, responsibilities, and standards across an organization. These policies encourage everyone to use data in the same way, resulting in more reliable data over time.
b. Implement data validation methods – Ensuring data is validated before entering a database is crucial, leading to fewer inaccuracies. For instance, checking the e-mail address, age etc.
c. Establish data Quality training – Implementing data quality training such as internal workshops or seminar covering topics like data error detection, or data collection practices, would help team members regulate high data quality standards.
d. Implement up-to-date documentation – Documentation of data collection, usage, and how it is processed helps people understand the background of data. Thus, helping everybody on the same page and avoiding unwanted conclusions.
“An active data quality initiative with automated infrastructure and tools is the highest maturity level that enables organizations to utilize data assets most effectively (check out Precience Data Sentinel, a Data Quality solution available on AWS Marketplace and Microsoft Azure Marketplace). Read More”
2. Lack of In-house expertise
For a successful AI implementation lack of in-house experts is a major hindrance. Despite the high demand for AI, many companies are struggling to find the right AI skilled talents. A survey indicated that 81% of IT professionals believe they could use AI, but only 12% people have experience working with AI.
To overcome this, organizations must invest in AI training, collaboration with experts, AI-focused workshops, access to online courses and certifications. Additionally, to begin with preliminary initiatives like pilot projects and AI tools would help in the initial phase of AI implementation.
“A Concerted effort to develop AI literacy across the organization and align AI as a key component in the learning plan is needed to develop AI competency across the enterprise. Reference taken.”
3. Outdated Infrastructure
According to a survey, by Cloudera, 90% of respondents said they are implementing AI but are struggling with outdated infrastructure. With the expanding nature of AI and processing large data sets, it needs a modern infrastructure with high computing capabilities.
Considering as the first step to implement AI, organizations must think of upgrading the IT infrastructure, which involves,
Maintaining high networks – To process large volume of data quickly and efficiently in the AI ecosystem, high-bandwidth, faster networks are necessary.
Implement scalable data storage – To handle growing data, organizations should scale their data storage system, by either adopting a cloud-based data solution, which offers flexibility and scalability, or on-premises data storage (physical warehouse), which provides reliability but is less flexible.
4. High Implementation Costs
According to Clutch.Co, the cost of both AI development and implementation may vary from $6000 to $3,00,000 in 2024. The cost for implementing AI depends on various factors such as initial investment, AI model development, integration and customization, long term maintenance, project complexity etc. Let’s look into some of these factors below.
Initial Investment – Factors influencing initial investment includes, acquiring infrastructure, data acquisition, and an AI platform, etc. For instance, AI implementation cost for fintech platforms can range from $20,000 to $1,000,000, depending upon the infrastructure, project complexity and other requirements of the industry.
AI integration & customization – Developing an AI solution and customizing it according to the organization’s needs can cost. The factor that makes AI development expensive or cheap is the decision to build it in-house or outsource. In-house AI development requires experts to build a highly sustainable solution, but time-consuming and expensive. On the other hand, outsourcing AI development would be much cheaper.
Project Complexity – More complex projects will generally cost the most for development and maintenance. Whereas simple AI solutions cost around $5,000. More complex projects can exceed $5,00,000. Hence, it would be beneficial for organizations to start with pilot projects and then expand.
Data Training Cost – The right quality data is of utmost importance for training AI models. Hence, collecting, profiling, and cleaning these large data sets would be more expensive.
5. Data Privacy & Concerns
Data privacy should be the top priority measure while considering AI implementation. Maintaining data privacy should be determined by an organization of what, how and in what way data needs to be shared or communicated to others. Today, it has become a necessity for organizations to prioritize the security of AI applications, as data is more prone to risk in the AI landscape.
These privacy challenges include data exploitation, biassed data, surveillance and monitoring, lack of transparency and so on. These challenges can be tackled by implementing data governance policies, abiding by data regulatory frameworks such as, General Data Protection Regulation(GDPR), the algorithmic accountability act, The california consumer privacy act etc. Additionally, organizations must conduct internal sessions on data privacy/security measures to create awareness.
Final Take
Understanding the necessity of AI implementation for businesses is essential, but it is equally important to recognize and address the challenges that come hand-in-hand. AI has the potential to generate significant profits for the organization.
Since AI is at the forefront today, adopting and upgrading to an AI space, irrespective of AI’s pros and cons, is crucial. This would not only help companies grow but also prepare them for the next technological evolution.
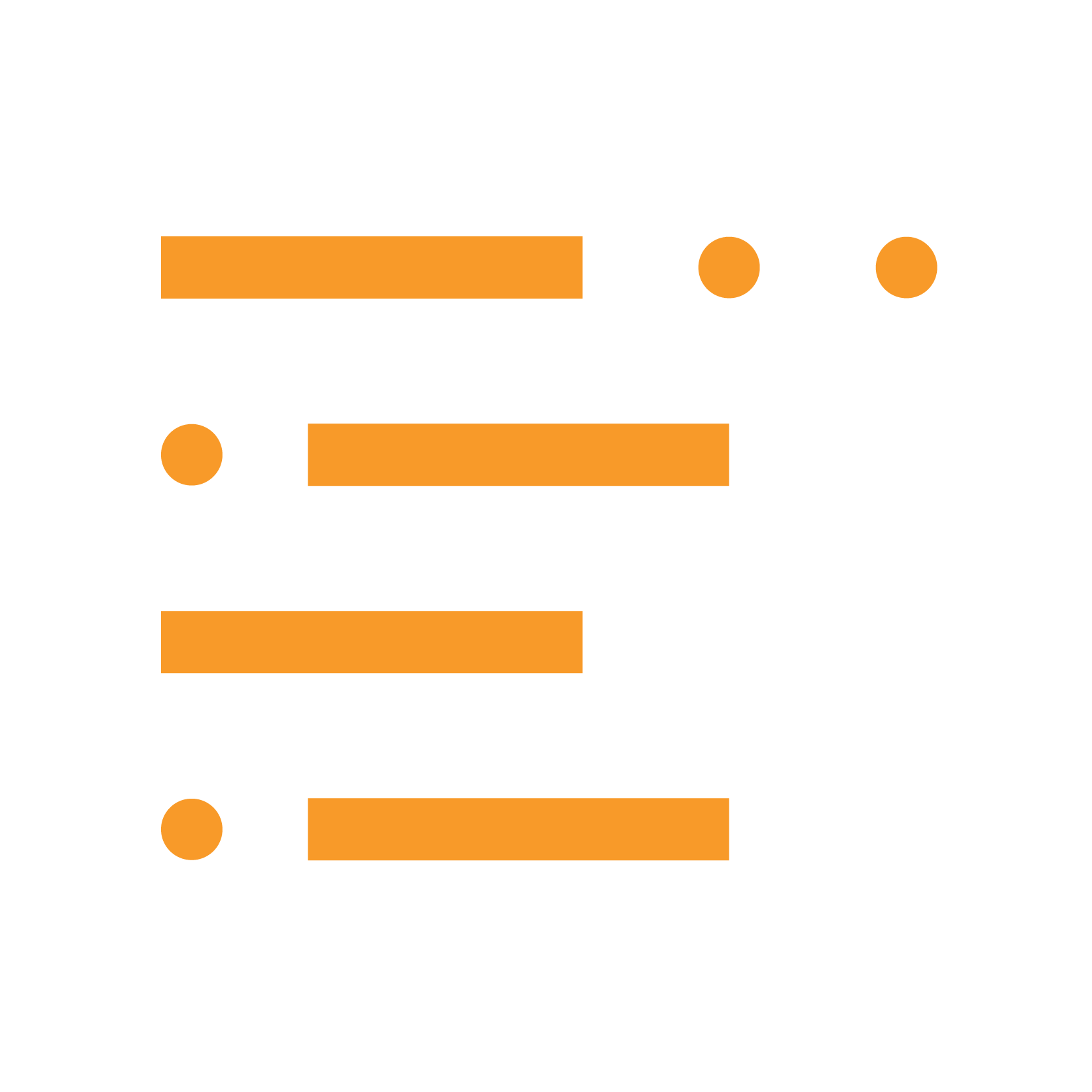
Prescience Team