May 7, 2025
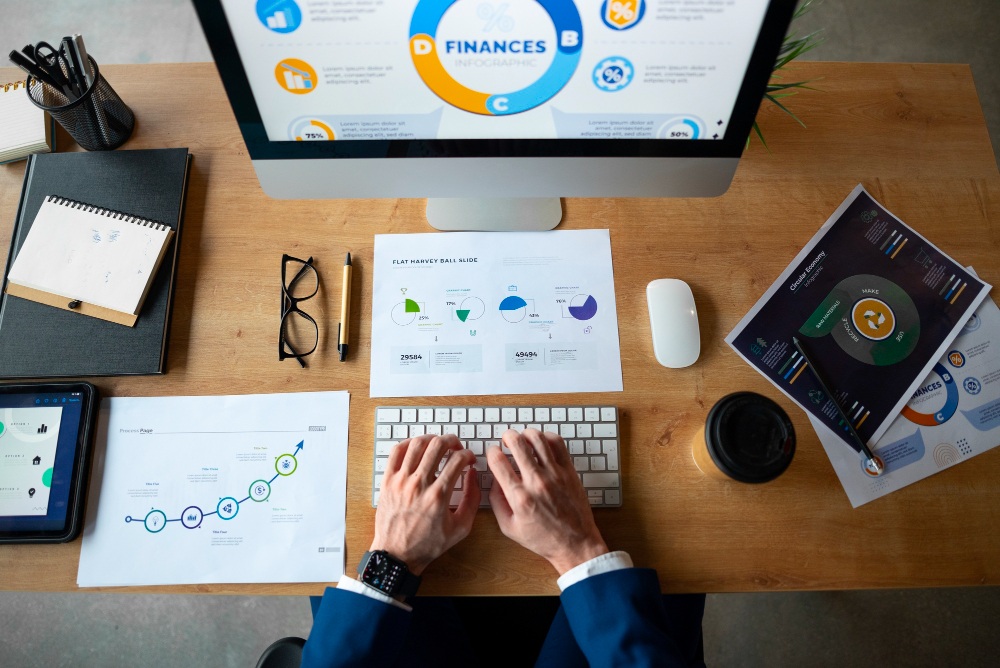
The company is one of the world’s leading strategy consulting firms and has a presence in over 50 countries.
THE CHALLENGE
The company was responsible for providing financial and technical consulting services to one of India’s largest public sector banks which has a customer base of over 500 million users. This bank is the market leader in personal loans, education loans, agriculture gold loans and loans to Self Help Groups (SHGs). The bank has a cumulative loan book of Rs 40.67 trillion. The bank’s risk management team did not have a comprehensive solution that provided a risk assessment analysis of loans taken by High Net Worth Individuals (HNIs) for amounts of Rs 50 million and above.
Based on this, the company designed a framework for implementing a series of advanced risk assessment Machine Learning (ML) models for the different market segments that the bank’s HNIs belonged to. Their proposed solution also leveraged the expertise of one of India’s leading credit bureaus. This framework leveraged historical data as well as insights from various financial statements, corresponding to each HNI that took a large loan.
The company required a data analytics partner to develop, test and roll out these ML models, while also benchmarking the results of the risk assessment framework against that of the credit bureau solution. Additionally, the partner had to generate millions of rows of synthetic data to test the output of each proposed ML model.
THE SOLUTION
The Prescience team analysed the proposed risk assessment framework and the different data sets that were available. Certain gaps in the designed ML models, proposed testing process, scoring methodology and risk grades were identified and the team recommended changes to the same. The HNIs were split into different segments based on the loan size – Rs 50 million to Rs 200 million, Rs 200 million to Rs 500 million, Rs 500 million to Rs 2.5 billion, and Rs 2.5 billion onwards.
In total, 23 different decision tree and logistical relations ML models were implemented for the different combinations of segments and loan sizes. These ML models covered the services, manufacturing, trading, banking, commercial, engineering, construction and non-bank financial institution markets.
The risk scoring process involved the extraction of key variables from the historical data, as well as supporting data sources such as stock market prices, risk ratings from the credit bureau solution etc. These ML models generated a corresponding risk assessment score, and using the ensemble methodology, each model was assigned a different weightage to arrive at the risk grade that the HNI would be assigned to. The solution overlaid grade wise risk calculations on these, to arrive at the final risk assessment score for that HNI.
Our team was also responsible for creating millions of rows of synthetic data that were used to test each of the 23 ML models, thereby ensuring the highest accuracy levels for each use case. In total, the risk assessment solution was tested for over 1.3 million different accounts with the synthetic data provided by the Prescience team. The final output of this new risk assessment solution was compared against the credit scores provided by the credit bureau solution.
The different technologies used for this engagement included,
- PyCharm
- Microsoft Excel
- Microsoft Visual Studio
THE IMPACT
With this new risk assessment solution, the bank has clear visibility into the probability of default for each HNI in each loan segment. This solution provides their risk management team with early warnings of potential loan defaults, based on which the loan disbursement officials can decide whether or not to sanction further loans to these HNIs in the coming months. The solution also provides the senior management with details on potential long term stresses in the lending division.