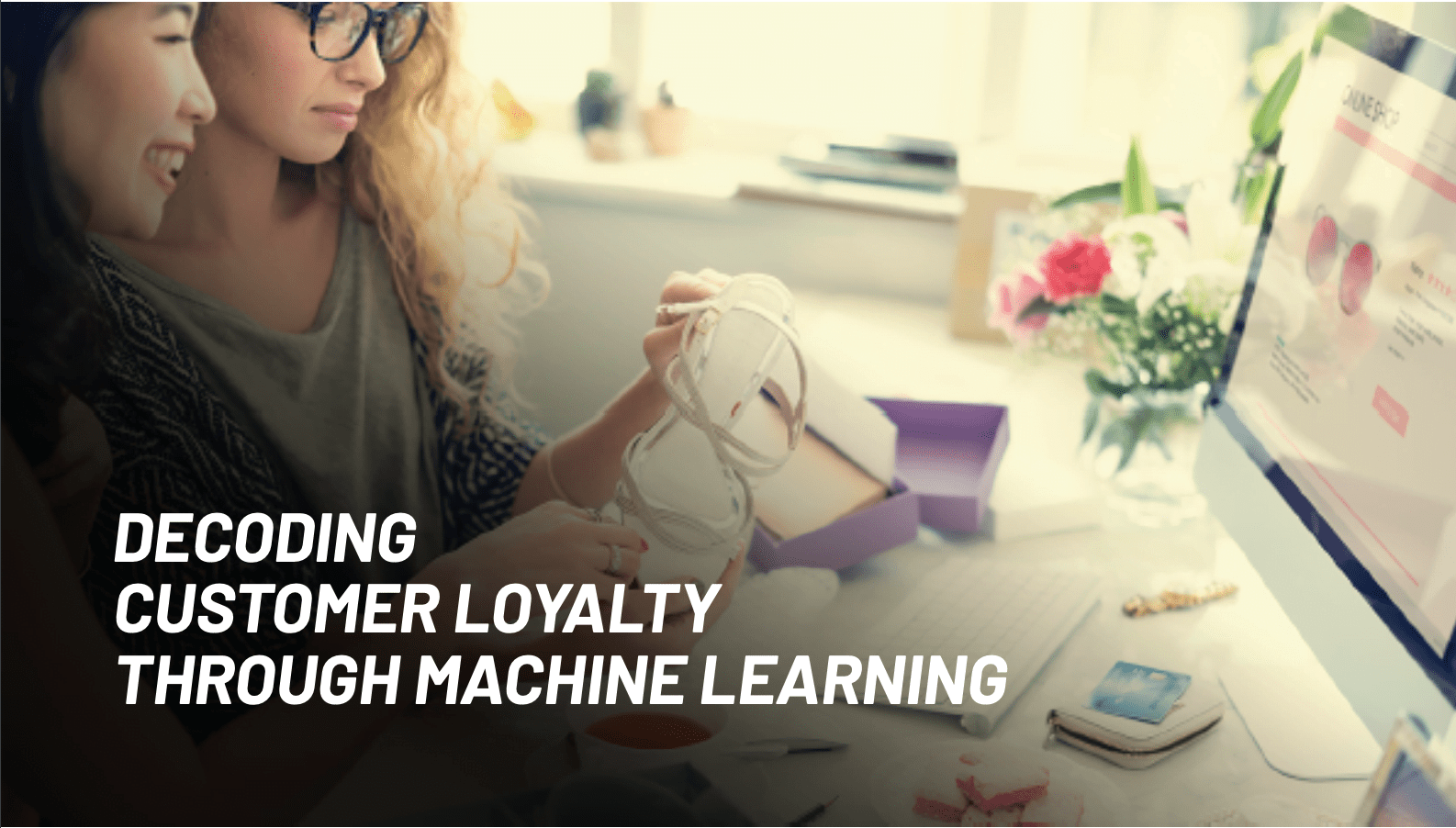
The company runs a large online auction platform and shopping website that connects buyers and sellers in over 190 countries. Their website has millions of sellers with 1.9 billion global listings and over 132 million active buyers.
THE CHALLENGE
The company earns its revenue primarily through fees collected on its marketplace sales, which includes the payment processing fees and first-party advertising charges. In order to increase the total volume of customer traffic and sales on their platform, the company uses an external vendor to offer a loyalty program for every buyer who is a resident of the United Kingdom (UK). For regular transactions, buyers earn 1 loyalty point for every £1 marketplace purchase, not including postage, packaging charges or any taxes. During special promotions, buyers can get additional bonus loyalty points or multiples of the loyalty points, of what they normally would. As buyers accumulate these loyalty points, they have two options to redeem them. Buyers can convert these loyalty points to vouchers, which can be used for subsequent online purchases on the same marketplace. Alternatively, buyers can redeem these vouchers with other affiliate partners of the loyalty program provider. In such cases, the company reimburses the cost of these redeemed points to the loyalty program provider. Buyers do not have the option of encashing loyalty points.
The company’s business teams did not have the required Key Performance Indicators (KPIs) to understand how much of incremental sales and revenue, this loyalty program was bringing to their UK business operations. In the absence of this information, the business teams used pre-defined tests and control groups to assess the loyalty process. However, the company believed that this approach was skewed by strong selection biases.
With insufficient and potentially incorrect data influencing their decision making, the company’s business users were unable optimise the ongoing loyalty program or accurately track their Return on Investment (RoI) of the program.
THE SOLUTION
The team of business analysts and data scientists from Prescience Decision Solutions had to first understand the likelihood of a particular set of buyers joining the loyalty program. Based on this, they could factor in their expected customer lifetime value (the predicted amount that customers will spend on goods or services over a period of time) and help the company predict the effectiveness of the existing loyalty program and make changes to it, as needed.
First, the team selected a sample data set of buyers who were located in the UK. Next, they developed a methodology that used a cohort approach based on customers linking their online shopping portal account to the company’s loyalty program. The team matched these details with available demographic and behavioural information, to minimize the possibility of selection bias.
Then, the team created a classifier with known user demographics data, transaction history and program enrolment status, to successfully predict the user’s propensity of enrolling into the loyalty program.
The overall approach leveraged machine learning models to match a non-loyalty user from the controlled user group with a high propensity for joining the loyalty program, to the nearest loyalty user from the test user group, with the same propensity of consumption. These matched results were then compared to identify the gap in their future purchase behaviour and overall customer lifetime value. Thus, the team was able to develop a synthetic control group to calculate the program’s incremental sales and revenue generation for each new customer added to the loyalty program.
The different machine learning models that were used included,
- Random Forest Classifier
- Feature Importance: Random Forest
Through this approach, the team was able to predict the future RoI of the program by matching the buyers who are part of the loyalty program with the ones who will be most likely to join the program, based on the above-mentioned buyer characteristics.
The different technologies used for this engagement included,
- R for the different machine learning models
- SQL for data preparation
THE IMPACT
The solution was able to predict the likelihood of different buyers joining the loyalty program with an accuracy of 83%. The company could also now determine the loyalty program’s RoI, the incremental revenue, as well as monthly and quarterly running costs. The solution also helped in assessing the value of new loyalty program customers, by comparing their Gross Merchandising Value (GMV) with that of existing loyalty buyers, for the same time period.